Zane Ball on Chuck Yates Needs A Job
0:20 You know, normally, 'cause you've listened to the podcast, which is why I asked you to come on, but normally the device I use is, hey, mom's listening, tell mom who you are. Mom actually knows
0:32 who you are. That's true. I saw her last night. Exactly. You were cool to fly in for that 'cause mom and dad, mom and dad really loves seeing you. I was happy to do it. I was just, when you
0:45 have a chance to see everybody together like that, and yeah, you just jump at it So, worked out. Wish I could have brought the family. I think I would love to see my kids. Yeah, no, that's
0:56 your man and your woman. They're not kids anymore. That's true, that's true. Scary stuff. So to level set for the audience, 60 year wedding anniversary, Charles and Sally Yates, and we had a
1:08 big party at the house, and mom and dad tried to buy that house back in the early '70s And so this is kind of like fulfilling mom's dream. by me buying that house and her getting to have the party
1:22 there. Well, that's kind of magical with those huge trees out front with the lights hanging down and everything. It was nice. Well done. It was really nice. So the other thing I'll level set
1:34 with the audience is Zane's of all the people that were contenders for the fifth Yates brother, I think you, you won the lifetime achievement award there will give you the title You know, Chris
1:49 Christenick may have had a little claim to it early days, but no, I think it's all yours. Awesome. So tell the audience the background, your background, because I know it. Yeah. So I'm an
2:03 engineer, went to Rice with you. That's where we all met out of school. I went to work for Intel and I've been an Intel for 28 years, done all kinds of different things that Intel from engineering
2:16 stuff to running business stuff. I led our desktop PC business for a number of years.
2:24 I lived in Taiwan for a couple of years. A lot of the IT world sort of goes through Taiwan, most of the world's computers and things are made by Taiwanese companies, and that was a huge experience
2:36 for me. I spent also a couple of years as Intel was trying to build a silicon foundry business. So that's where we manufactured chips for other companies, which is kind of the usual way the
2:46 industry works, Intel's using a little bit unique in the industry these days, that we still like both design and manufacture our own chips. And after doing that for a little while, I went to our
2:56 data center group, and I've been there for a little over 60 years. I managed our engineering and architecture teams, developing new Xeon servers, and work closely with the big cloud service
3:09 providers, and big OEMs like Dell and Amazon, and companies like that to build computer infrastructure.
3:20 Intel has traditionally done a lot with is industry leadership things, like building standards. And my team did a lot of that kind of work. So when you use like a USB port, you know, we kind of
3:31 started that and a lot of the work done there. And
3:35 you know, we were active in creating ethernet and PSYX press and all these kind of technologies. And as we've moved into this kind of big data center world, we do a lot of development of new
3:47 standards, you know, working closely with the industry. And so that's been an exciting part of the job. But I just got a new job. Oh really? Yeah, so two months ago, we did a big, you know,
3:60 big reshuffling and so I'm now, I've moved back to the business side. So I'm leading product management for our server and AI products. So like the Xeon processors that are Goudy AI accelerators
4:15 and stuff like that, so.
4:18 You're cool to come on the podcast, 'cause I wanna hear everything about data centers and AI for, because at the end of the day, that's gonna take a lot of power. I mean, you know these stats
4:33 better than I do, and I've said it a lot on the podcast, so the audience knows it, but, you know, 1950 to 2000 electricity usage in America was up 15 acts, right? I mean, you just built a lot
4:38 of
4:46 really cool stuff that ran off electricity, AC dishwashers, the computer, et cetera, you know, 2000 to, let's call it, almost today, electricity usage in America was pretty flat, and it was
5:04 'cause the machines got a lot more efficient and all that, and now everybody's talking about, you know, 2045, we'll be using 3X electricity, All of this is data center-driven, AI-driven type.
5:18 stuff. So don't forget the EVs in the
5:26 EVs. Yes. Yeah, the EVs. Although I don't know that that's going to take a we'll debate EVs. I think the people that are forecasting that big increase are assuming a big chunk for EVs too. Yeah.
5:35 But data center and AI is a big part of that forecast. Absolutely. Yeah. So how are you going to power all that? Because I mean, I feel like where we are is, you know, I was listening to a
5:47 podcast with the head of MISO, which is the electric district, kind of Midwest. And he said there, they used to have 15 excess generating capacity. They may have 2 now. It feels like we're all
6:02 tapped out. So how are we going to power all that? I think it's a great, great question. I
6:11 think there's a few things to think about about, you know, I'm really interested in that. period we just finished. Like you said, we're, so how did we do it before, right? You know, and in IT
6:22 and, you know, just the last 10 years we went through this cloud computing revolution and computing capability, you know, expanded by about 500 in terms of, you know, the amount of capacity,
6:36 especially in cloud computing and, but electricity consumption by data centers actually didn't grow very much. It's like single digits. Yeah, the the stat I've heard is computing power in the
6:47 cloud was up 10x over the last decade, but electric usage for all of it was up maybe 10. So basically, what you're saying. And you know, there's some researchers that have, you know, gone and
6:60 tried to break that down and sort of how we got to where we are, because I think it's good to understand what worked well so we can think about what might work well in the future. And there were
7:09 really three pieces to that story. One is like just Moore's law, right? Chips get dramatically better. we're able to put dramatically more computing power in one chip and therefore one server can
7:23 do the work of, you know, wait five, six, seven years and one server will do the work of 10, you know, and so it does get dramatically more energy efficient. But that alone doesn't explain the
7:37 success. The other big change in the world was this kind of movement into these very large scale data center, how we go on hyperscale data centers. And you know, you'll see there's a lot of them
7:48 in Oregon where I live. Oregon's kind of a great place for this kind of thing of cheap green electricity. You've got great fiber optic infrastructure. You got the property tax laws. All works
8:01 together to make this a great place. But you see these facilities and you know, it makes like the biggest cost code you've ever seen look like a small box And there'll be, you know, a substation
8:13 on one side of the building and there'll be all these cooling towers on the other side. you know, there's a big industrial scale, it's like a digital factory,
8:22 but those kinds of facilities are far more efficient. You know, so the, one of the metrics that he's in is called PUE power usage effectiveness. So how much power is sort of wasted that isn't
8:33 actually computing something, you know, in the facilities and those numbers have improved, you know, dramatically. So that's kind of one piece. A second piece was a shift in technology called
8:43 virtualization And virtualization is where we get back to point one real quick. Just that more efficient is that in effect cooling better? I mean, is that the big driver is a big part of it, but
8:58 delivering the power is also a big part of it. So you waste a fair amount of electricity and how you get the electricity to the device. Okay. Because you have to go through various power
9:07 conversions and distribution. And so there's all kinds of losses. And, and then there's also. when you manage at a very large, you know, fleet level, you're able to do clever things to utilize
9:22 the capability, you know, more and more efficiently. So it works, but cooling is a big part of it. Like a lot of the, well, different parts of the world have different climates. So you get
9:35 different solutions in different places, but like in
9:40 Eastern Oregon, you know, one of the benefits is a very dry climate. And so if you have access to a water source, you evaporate water. And so they may consume a great deal of water, but they can
9:49 be very energy efficient. So you think like a swamp cooler in your house is much more efficient than an air conditioner. But if you live in Houston, you're not gonna get very far with the swamp,
9:59 right? But yeah, but things like that. And there's a lot of things in the future that can make that also a lot more efficient. Gotcha. All right, back to number two. Yeah, so number two is a
10:12 big change was called virtualization. that maybe the easiest way to describe that is instead of having you know you sort of separate the software version of a computer from the hardware version of a
10:26 computer so traditionally like I think of your laptop you know there's windows is running your laptop or something and that's running on a particular microprocessor or the particular chunk
10:36 of memory
10:38 but virtualization we're able to create a digital version of the computer on the computer so I don't know if that makes it makes any sense so it's a virtual version of a a compute instance and so what
10:48 you're interacting with in the cloud is a virtual computer not actually the direct you know metal on the on the chip itself it's a software almost like an emulation of a computer and learning how to
11:00 do that very well and very efficiently was a big technology development you know 20 years ago and that became widely deployed and that lets when you can decouple the usage of the computer from the
11:11 specific one you can manage the resources way more efficiently. You know, I can have more than one computer on a server. I can move them around if I need to do maintenance. I can do all kinds of
11:22 magic. And so you don't need nearly as much hardware if you can efficiently utilize the resources with this virtualization technology. So this turned into a big deal. And like almost all of the
11:34 most, all of the digital services are delivered that way today. There are applications where people do what you'll hear in the industry, people talk about bare metal servers and bare metal is when
11:44 you don't necessarily use the virtualization, you like rent the actual metal, the actual hardware from the provider. And then you do with it what you want, which may still include virtualization,
11:53 by the way. But most
11:56 services are delivered through these virtualization. And it's been a massive, you know, sea change in things. So between those three things, this, you know, innovative software technology,
12:06 virtualization, after and hardware. There were things we had to do in the chip to make that work
12:13 this big, hyper-scale infrastructure, chips getting better, when you put those three things together, that's why you were able to grow so incredibly much capability without really growing
12:23 electricity consumption all that much. So that's like a big success story. But then, you know, now the world changed, you know, where AI is a far more computationally intensive activity. And so
12:38 you're doing things on a
12:41 much bigger scale, much more complex machines You know, and then consuming a great deal of power as you process just a huge amount of data. And so now we are seeing this kind of inflection point
12:55 and the question is kind of what happens next, right? And I think there are some analogies, right? I think as electricity becomes scarce, people have to become smarter about it. And I think as
13:06 the economic demands on more electricity becomes stronger, you know, people have to respond to that with more, more, more capacity. And then there will be some, I think, market forces that
13:18 cause that to bounce out over time. And I think one of the things we don't know is what is the economic value of large AI models? So they're amazing. I was playing with GPT-O this week that came
13:34 out and it's amazingly cool what it does. But exactly how are people going to make money with it? How much money are they going to make with these technologies and then how much are they willing to
13:42 pay for the power to drive them? But so you see big players in industry investing. Significantly, you see utilities, I think starting to think about investing in AI. You see things globally
13:57 happening
13:59 in the Middle East and other places where people are thinking about this equation of industrial level AI farms and coupling that with investments in electricity generation together some providers have
14:13 even looked at these. small scale nuclear power plants. So if maybe if the one vision might be that if the cloud computing era was marked by these giant warehouses with a substation on one side,
14:25 maybe the future instead of a 100 megawatt facility with a substation, it's a 3 gigawatt facility with its own nuclear power plant. Yeah. Yeah. That's not true. I don't
14:37 know. Bigger infrastructure than that has been built in other industries, so it's not impossible to imagine If there is, you know, if there is demand for it, and if you think about, I don't know,
14:47 I don't know what a nuclear power plant costs, but you know, it's probably10, 20 billion, but the capital expense expenditures today is, and some of these, you know, big, big players, you
14:57 know, are on that order already, right? So if this market truly, if we're at the beginning of a big expansion, you know, you can imagine there's enough capital room, if you will, for some,
15:07 some big investments, and that'll be a global, a global thing.
15:13 The way, so I'm going to give a speech next week to a company that's a gas storage company. So they have their user conference and it's in a bunch of natural gas players. And the whole point of my
15:26 speech is gonna be, hey guys, you gotta stop thinking about selling natural gas and start thinking about selling electrons in some way, shape or form, because of what you just said. I mean, if
15:39 tech decides we wanna go nuclear, and there are a whole host of reasons on why you'd wanna do it. I mean, the cost is all regulatory. And if anybody has the ends to change regulatory stuff in DC,
15:53 it's big tech, right? And so if they come up with a design for a small nuclear device of some sort and get regulations put in their favorite, you can do it pretty cheap. I mean, the French did it
16:08 pretty cheap in the 70s and their electricity costs. are on average or below average for Europe. So they can do new, and that was the French back in the '70s, were much better at manufacturing
16:21 today, and
16:23 we're not the French.
16:26 But no, so I think the natural gas provider has to figure out some way to in effect, be the crack dealer and give the crack and get technology addicted to natural gas-powered electricity or else
16:42 they may lose out to it, to exactly the setup you just said, nuclear reactor right here, power this. Yeah, I'll throw another thing out for you to think about 'cause there's maybe two halves to
16:54 the AI equation. AI computing is kind of a different paradigm than traditional computing, because
17:04 you train a model and then you use a model. And those are two different activities And, um, Normal computing, nutritional computing, it's happening in real time. You ask a computer to do
17:16 something with a computer program, it works on it, it doesn't calculations, and gives you an answer. And how fast that answer comes back is important to you and getting that answer to your end
17:24 user, like when you're using an app on your phone, right? You're very interested in getting an instantaneous response. That's not happening on your phone, it's happening in the cloud somewhere.
17:36 With AI computing, when you make the model, you train the model, that's a very slow and expensive, laborious process, but then using the model is separated. So that being kind of two computing
17:48 stuff. So the training part of the model can actually happen kind of anywhere at any time. A big model might take weeks or months to train, and you might train that model in a totally different
18:02 geography than where you use the model. And if the computer crashes more often, it isn't that big a deal, or you had to slow it down because. of how our costs go up and down, you might be able to
18:13 manage that. And in the using of the model, we call it inference, the inference part of the model is where you already built the model and now you take that model and put it in computers that are
18:23 much closer to where the users are gonna be. And so then you do care about the quality of service and how quickly things come back. So this training step is really interesting because it doesn't
18:33 have to be in any particular place. Yeah, it can be next to the power plant It could be, well, other than this regulation becomes a big deal if you think about the data sovereignty laws. So the
18:45 data needs to be somewhere and there's very large amounts of data required to train a big model. And so you need to be where the data is, you need to be where the power is cheap. And that's kind of
18:57 interesting. So what's gonna happen in the Middle East? What's going to happen in China? What's gonna happen in Europe or the United States might be strongly affected by utility policies, energy
19:08 policies and data sovereignty policies and data policies and energy policies may need to go together to see where you're going to do this kind of all-important training stuff. But if you can't get
19:21 the end product to the user through the inference stuff, which might also have regulatory constraints at some point, then training it some far off location may not make sense. Or maybe for nuclear
19:35 power, you put that in some remote location, or if you're. Anyway, it's a different paradigm than maybe the infrastructure that we built in the past because of that. And it'll be interesting
19:48 where it goes. No,
19:52 I mean, I don't know which ones are which, but I've read about in Japan, you have certain rules on how you're allowed to train the model, what's fair use, what's not, so far in America. We've
20:06 been pretty liberal about fair use doctrine and. and what we can train the model on. But if you make the analogy to Bitcoin mining, you're right, you just put it anywhere. I mean, 'cause you see
20:22 the miners just chase low. Oh, that's a little bit low. And I think it's more than an analogy. I think kind of the folks that have invested in Bitcoin mining are probably also going to invest in
20:34 AI farms as well. They're just GPU farms in a sense I'm figuring out a cheap economic way to operate a big fleet of GPUs. I mean, I think there are differences, but there's some definitely
20:47 similarities there. Yeah, then you're right. And then when
20:53 XYZ state or maybe even different country has the most liberal training rules out there, the NGs are all figure out, Well, you can't use that language model when you're in the state of New York.
21:12 Or something to that effect, yeah. You could imagine it getting pretty complicated. Yeah, that's wild. So now we've talked the past, put on, look into your crystal ball. What are we gonna see
21:27 happening on that front? Do we have Moore's law still going? I mean, it'll just keep getting better. It'll keep me more efficient. And maybe we're overblowing estimates for electricity or even
21:42 with Moore's law going. There's gonna be so much demand for AI. I mean, I think when your best friend runs off with your girlfriend, you'd like Apple Music to just start playing, you know,
21:53 whatever by Hank Williams Jr. without thinking about it. But yeah. Well, it's a good question. I think first to Moore's law, sometimes we wrap up a lot in that word And literally, Moore's Law
22:07 just speaks to - doubling the number of transistors in a chip every couple of years. And, you know, I think at least through 2030, you know, that's, you know, you can see a pretty clear path.
22:19 You know, we've talked about a one trillion transistor device by 2030. You know, it gets, the technology gets increasingly exotic as you do that. But because there's such demand and because the
22:32 impact of improvements in semiconductors drives such important improvements, even if the improvements started to slow down, the value of them, I think, is you're scaling across so much of the
22:42 economy, it gets higher and higher. So I think you're willing to keep investing. And maybe it isn't delivering their returns of 30 years ago, where you had Moore's Law in terms of Moore
22:51 transistors. But you also had another thing that us wonky people talk about called Denard scaling. And Denard, I think it was IBM researcher.
23:02 And he died just like a couple of weeks ago by
23:05 the way, kind of, more is a lot. Him and Gordon Moore die. I kind of gonna hear of each other.
23:12 But Denard scaling was recognizing that not only when the devices got smaller, they ran faster and consumed less power. And so you got like this amazing win, right? That every generation of
23:20 technology, not only did you get more transistors, but the transistors were half the power and 70 faster. Yeah, and that scaling has petered out, you know, over time. So you don't get these
23:34 outsized gains that you got 30 years ago, but you still get important gains. And that's why we spend even more than we ever spent before and build even more elaborate factories to do even more
23:45 exotic technologies because the value of it is still very strong. But there's some things that kind of cut against Moore's law saving you here. One thing is that, especially in AI,
23:59 an increasing amount of the power is spent moving the data around So you have on a GPU, you have this high bandwidth memory that's, you know. trying to get as much data into the chip, get it out,
24:13 and then you have to connect those devices together with very high-speed data network. And then those devices have to be connected across rack-to-rack, to rack through these very high-speed networks.
24:23 And all of that data movement, and drives a lot of power consumption. So the amount of the power that's consumed moving the data around just gets bigger and bigger. So even if the computing part of
24:34 the power consumption gets better and better, you've got to bring the cost of moving the data around, or you have to find a more clever way to do it. So maybe you don't have to move the data around
24:42 as much. You know, so there's a lot of research in those different kinds of questions. But I don't think, just like in the cloud computing here, Moore's Law alone didn't save you. And I don't
24:51 think in the next generation of AI, Moore's Law is gonna save you either. But it's definitely essential. If you're not building more capable devices, you're not gonna be able to keep improving.
25:03 Now, one of the big debates, and this is kind of a crystal ball, And oftentimes not only is it one or the other, but maybe sometimes many things can be true, but there's a little bit of a debate
25:13 between what's gonna happen with the size of AI models. And so, some people believe that smaller models are gonna capture more and more of the market because there's so much cheaper to operate,
25:25 they consume less power. And then others are like, no, we're just gonna build just every year the size of the model has been going up 10X, by the way. That's been the trip. And so, a chat GBT
25:37 or whatever, maybe a trillion element model, and the next year it'll be 10 trillion and 100 trillion, and how long is that growth gonna be? And maybe these uber powerful models can do every
25:47 possible task. That's one feature. The small model people believe, okay, I've got a good quality, smaller models. It's only 70 billion or 100 billion. Like meta has open source model, Coloma 3,
25:60 and anybody can use that open source model. Well, if you couple the smaller model, but with very focused data. you can also get a very good result. So I mean, if I'm building an application, I
26:12 don't need it to do everything under the sun in the way that I might use chat GPT. You know, I can ask chat GPT anything for poetry to history to, you know, quantum mechanics. And, you know,
26:24 but if I'm building a business, maybe I just, I'm Spotify. I just wanna, you know, I just wanna, I just wanna select music and I have all this data. And can I apply all my data that's my
26:35 private data? And can I just use kind of a standard model and work those two together? And there's two ways to do that. One is you can fine tune the model, which means you do additional training
26:46 of an existing model, but you train it on very task specific data. Imagine your law, maybe your finance department and you train it on your finance data so that you have a, you have something that
26:57 can build financial reports are very accurate. You don't need necessarily the giant model to do that You just need to tune, you know, a less capable model with the right data.
27:09 And so that's one path. And another thing that's even simpler is a technology called RAG or retrieval augmented generation. And in the RAG
27:20 use case, you just bring the data with the model. You don't change the model, just use the model, but with lots of specific data. So imagine it being like incredibly elaborate prompt engineering,
27:30 where you're just feeding the model, your company's private data. And then now you're gonna get a far more accurate result As long as you just keep it to what, keep it within the domain of where
27:41 your data is. And so then you did all this work, you got something that's pretty good result, but you didn't have to, you didn't need a nuclear power plant, you know, necessarily to train that
27:50 model, right? So. You know, it's wild, you bring that up because literally what we've done around here, digital wildcatters over the last six to nine months, is we looked up and realized we had
28:03 created all of this energy specific content. we went out, we built a RAG model. Okay. You've actually done it? Yeah, well, I'm still an idiot. This way above my pay grade in terms of learning
28:14 this stuff, but when we make a query on collide GPT, we've got four language models on any given day. We'll use whichever one is the cheapest, whichever one's performing the best, whether that's
28:29 llama, whether that's chat GPT or whatever. And then we get four answers, and then we use, I think we're using chat GPT right now to choose the best answer. And it's hitting our database of
28:42 energy specific stuff, 'cause we've got all our content in there, we've gone out and I think we've got now 3, 000 videos in there. We've scanned in
28:55 text books, et cetera. You name it on third-party curated energy stuff. And about, you're right, 80 of the time, If you ask an energy specific question to - collide GPT, you get a better answer
29:08 than chat GPT, just because it's hitting this database of energy stuff. And we've tuned it to give preferences there. And you protected your data. It's yours. That's your, that's your content
29:18 and we value add. You don't want to give that away to train someone else's mega model. You want to keep that. Exactly. Differentiation. So exactly. So that's why potentially in the future,
29:27 we're ripping down all our podcasts off YouTube where people can go scrape and you're only going to be able to get that through collide because there you go. And so there's an energy dimension to
29:39 that exact thing you just did. Right. So you open up the possibility of getting the result you need without continuing to grow the model size. You know, and so therefore. And this is interesting
29:50 too. Cause we've also have the belief that ultimately no one's going to go to collide GPT and say something like, can I put friction reducer in my mud? and if you get the answer, yes. Okay, go
30:05 ahead boys.
30:07 Actually, what we think people in the energy business are gonna wanna see, is they're gonna wanna
30:15 see, yes, you can do it, XYZ, here's an answer. Oh, by the way, this comes from this SPE paper. 'Cause the engineer is gonna wanna go, ah, there's this technical paper on that. That's an
30:26 idea I didn't think of, but now I'm gonna actually go to some more. We're not just gonna. Yeah, so we almost call it like advanced search is gonna be more important in energy 'Cause at the end of
30:36 the day, just with health and safety concerns and the expense of drilling well and the like size, Exxon's never gonna have artificial intelligence trained
30:52 on something they randomly found out on the internet. It's gonna be Exxon specific data if they start using that stuff. So it's been fascinating to see, I'm jokingly running around telling
31:04 everybody,
31:06 Podcast bros to a legit AI to software. Just put dot AI in your, In the funders. Yeah, it works better, yeah. Exactly, but now you're right. You're right to say that too. Does Zane Ball have
31:19 a belief on what shakes out in the future or you just pose to the question? Oh, I mostly humble and pose to the question. I know smart people that are very passionate about both sides of that. I
31:30 think there's definitely both are going to exist. I mean, so I think there's going to be a whole spectrum of solutions, but that from an energy perspective, it's like, well, what becomes
31:37 dominant, right? And I don't think people are going to slow down building mega models. I mean, I think they're going to just keep on going, right? I mean, we're in a, people are investing like
31:47 crazy. So what we see today is only, you know, the beginning of what you're going to see in a year and two years, you know, because the investments and the capital spending is off the chart. And
31:57 people are, people are racing to, you know, advance these capabilities and so they're not doing that because they don't believe that they're going to get something very special. special out of it,
32:07 but we'll find out. I think the part for me that's the biggest wildcard isn't so much the technology. I think people are going to build big models. They're going to do amazing things. But a little
32:17 bit like the birth of the internet, at the beginning, it was obvious to everyone that it was going to be big, but it really wasn't obvious exactly how the money was going to be made. And I think
32:27 there's some similarities here. I remember seeing my first web page in 1994, you know, and thinking, that's cool that's got to really going to be something. And you know, for the next two years,
32:37 every company in a world was putting up websites and, you know, they didn't know why they were putting a website, but they're all, they're all just doing it, right? And you know, it's not even
32:44 that different reading about like the railroads in the 1850s or whatever, people were just building railroads everywhere, you know, and they were right, you know, it was going to be big, but it
32:51 wasn't really clear how it was all going to work. And so, you know, it wasn't until, you know, you didn't get, you know, what Google until 1998 or you didn't, you know, you didn't get
33:01 Facebook till 2005,
33:05 You didn't really see how the industry was going to play out immediately, right? So, so I think a lot depends on that, you know? So these amazing models are going to be built. I assume they're
33:15 going to be pretty profoundly useful for stuff. How are they going to get paid for and how often are they going to be used? And I think that that will have, that will have some big energy
33:24 implications. So what are kind of other, you know, aside from, from energy usage, that, that was interesting, hadn't thought through the whole, if we've become a world of rag models versus
33:37 super models, what, what other things are going to potentially impact energy stuff? I think energy usage and AI is, is, I think market forces are very powerful, right? So energy gets scarce,
33:51 people are going to be very careful about how they use it. And
33:56 AI is pretty immature, you know, as amazing as it is I think the, to what degree of all the genius data scientists of the world really deeply thought through. the most energy efficient strategies
34:07 for training, or even in French, I'm sure there's innovation to be had, and if there's one thing, I think the energy industry teaches us is that
34:19 when market forces come into play really powerfully, people innovate. They come up with different ideas, and so I do think the idea that you just have some sort of unsolvable problem with energy
34:32 growth, either there'll be more innovation that gets us more energy, or there'll be more innovation that says we don't need as much energy. I think there'll be some combination of the two. So I'm
34:44 sure that these curves that project AI energy consumption are probably wrong. They're accurate in the sense if you do the math on where we're going and what the current paradigm is Those are good
34:57 forecasts made by smart people, but I just have to believe there's game-changing innovation that's going to bring that curve down to size. I don't think we're going to get back to this flat scenario
35:08 like we've been doing because I think it is a, it is a massively more computationally intensive thing, but I, I,
35:17 I'm incredibly optimistic that, you know, given the right boundary conditions, the engineers figure stuff out, then they, and they've done it again and again and again, and it'll just be
35:26 interesting to see this is a race between the energy industry engineers and the tech engineers. Yeah, who's going to make the biggest dent on that problem? I bet they're both going to make some
35:36 pretty big dent on that problem. Yeah, I think the issue in the energy business is we got beat up. I mean, the shale revolution for doubling oil production in the United States, natural gas
35:51 production, like I mean, we literally went in a decade from building things to import natural gas, because natural gas at15 an M to your point, innovation, Ben. We've converted all those places
36:06 to exporting natural hands now, because we've got so many of them. I think what I worry about though is, so the Shell Revolution did all this amazing stuff, but we lost a lot of money and we in
36:18 effect shrunk as an industry. And then we had COVID and we fired half the industry. And so the entrepreneurial spirit we used to have, at least part of it was, we had a whole lot of people. And
36:33 then number two was, we had a whole lot of capital access that we just don't have today, because we, uh, we, we incinerated capital there for a while. And then you put kind of the green issues,
36:46 overlaying, all this stuff. It's not uncertainty that you have to deal with in terms of climate change and what the future regulatory environment is going to be. And exactly. I think that that is
36:56 a really complicating factor. So if you, if your plan is to sunset all of your fossil fuel plants when they expire, but you don't have
37:04 you have to not only have sufficient renewables to replace that. You have to have sufficient renewables to account for this massive growth at the same time. It seems like a pretty tough circle to
37:15 somewhere. And we've never actually transitioned away from a fuel source. We burn more wood today on the planet than we've ever burned in our history. So, I mean, it's always been addition. It
37:26 hasn't ever been a transition away from something. So you put all that together I just worry about our ability to be entrepreneurial enough to make the dent in it. As you said, versus we're going
37:40 to seed that to technology. Because you guys still have a lot of capital behind you. You've got all the smart engineers, etc. And I worry in, this sounds bad. I'm making sound territorial. I
37:54 mean, at the end of the day, whoever does it, does it. But it just, I worry that it gets seeded to the technology companies. Maybe they become the source of capital. You know, you see them
38:03 investing and. like these micro nuclear weapons, you know, like they're, they're definitely taking a strong interest in understanding where their energy is going to come from, because that can
38:14 cap their business. In fact, I think even, even right now, people are thinking very, very carefully about how they're going to secure, how they're going to secure power, where they're going to
38:24 build those data centers, how they're going to, um, secure their place in the, in the future. And that's why they're not going to be passive. I wouldn't expect them to be super passive about it.
38:35 There's a lot of money at stake and they have a lot of capability to invest. Yeah. They don't, they, these companies haven't typically left their fate to, you know, to others. No, that's very
38:47 true. I mean, that's John Arnold, the world's greatest natural gas trader. That's why he's on the board of meta now. Cause Zuckerberg's like, we need to understand energy. So it's kind of crazy.
38:60 So kind of final thoughts. What else do we need to be thinking about? when it comes to AI data center's power.
39:09 I guess we covered all my big thoughts. I think
39:13 it's just going to be real interesting. You know, I think we can talk a lot today that I think there's a level of change and transformation going on that eclipses anything I've known in my 28 years
39:26 in the industry. So the opportunity for things to look very different and they look today in five years for different companies to come on the scene or leave the scene and
39:38 big differences in business models. I think the way it intersects, the way technology is now intersecting with energy and intersecting with geopolitics and
39:51 government action in many areas as well as data sovereignty or energy policy or whatnot, you know, all of those things are significantly complicating the world.
40:04 in the age of globalization, you know, in the industry. And I never would have thought that, you know, in the tech industry is a very global, the chip industry in particular is a very global
40:14 industry, right? You know, things are built in all kinds of parts of the world and IPs developed in all different kinds of parts of the world. And we've just had it free flowing across the planet
40:23 for the whole industry is structured that way. And now we're, you know, dealing with a very different world where, you know, things are shaping up to look different and the equations are more
40:35 complicated. I think we had the benefit in the last 20 years of just kind of having to do the engineering and finding the cheapest way to do things and to serve customers. And now you have to think
40:44 about, you know, a much more complicated world. Yeah, no, that is
40:50 crazy. The one thing, the one thing I'm probably going to spend a lot of time on on the podcast I bet over the next year is we have this running joke that if natural gas is going to be the fuel
41:02 source for all of this. Monahan's Texas, which is out in West Texas. And where do you want to go to Monahan's Texas? You know, my dad lives in Midland. Oh, that's right. You know where
41:10 Monahan's is. Well, they're going to have to upgrade from having the Ben against be the nicest restaurant. If they want to entertain Intel executives out in West Texas. So we're going to invest in
41:24 a chain of high-end restaurants in West Texas. So maybe when the high-end vegan restaurants open in West Texas, we'll know. There were no new restaurants There was somebody took a Silicon Valley
41:35 investor to
41:40 Schneider,
41:42 Texas, and they were at the Schneider Country Club, and supposedly the investor asked if there was a vegan menu, and they kind of stared at him and skews us, but anyway. I was just in Midland a
41:56 couple of weeks ago visiting my dad, and my brother was there too, And
42:01 we had Sunday lunch at night. Wall Street barn grill in downtown Midland and you know, I can only imagine the The deals that have changed the industry that have been that have been struck at the
42:13 very table. We saw that That's how you that was that was fun used to sell you the stake for the price of oil Was fagging the day so Well, Zane you were cool to come on to do this and even more
42:25 importantly you were cool to come see mom and dad last night It's been a pleasure.
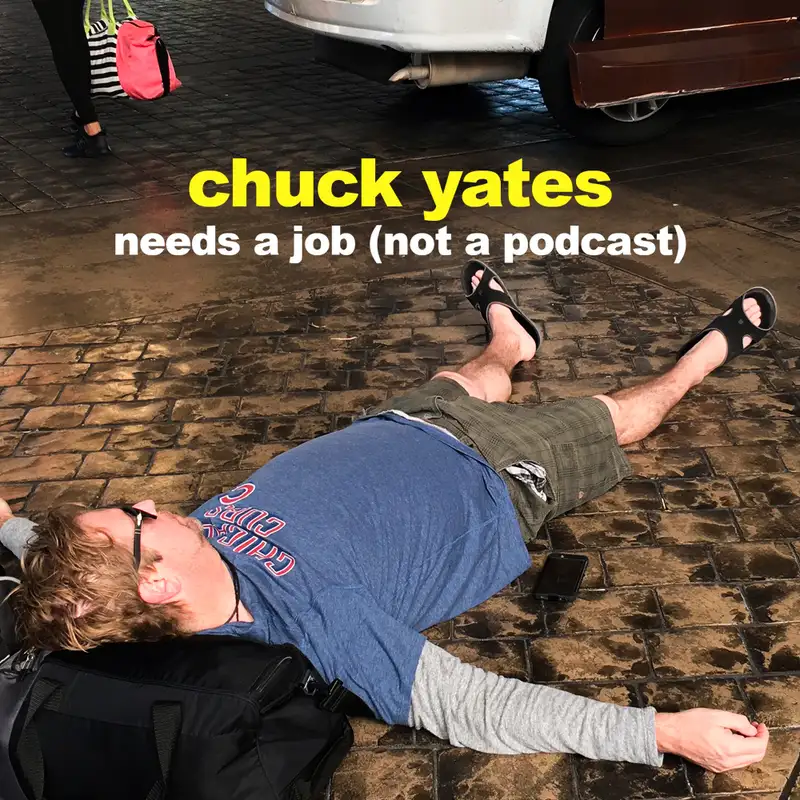